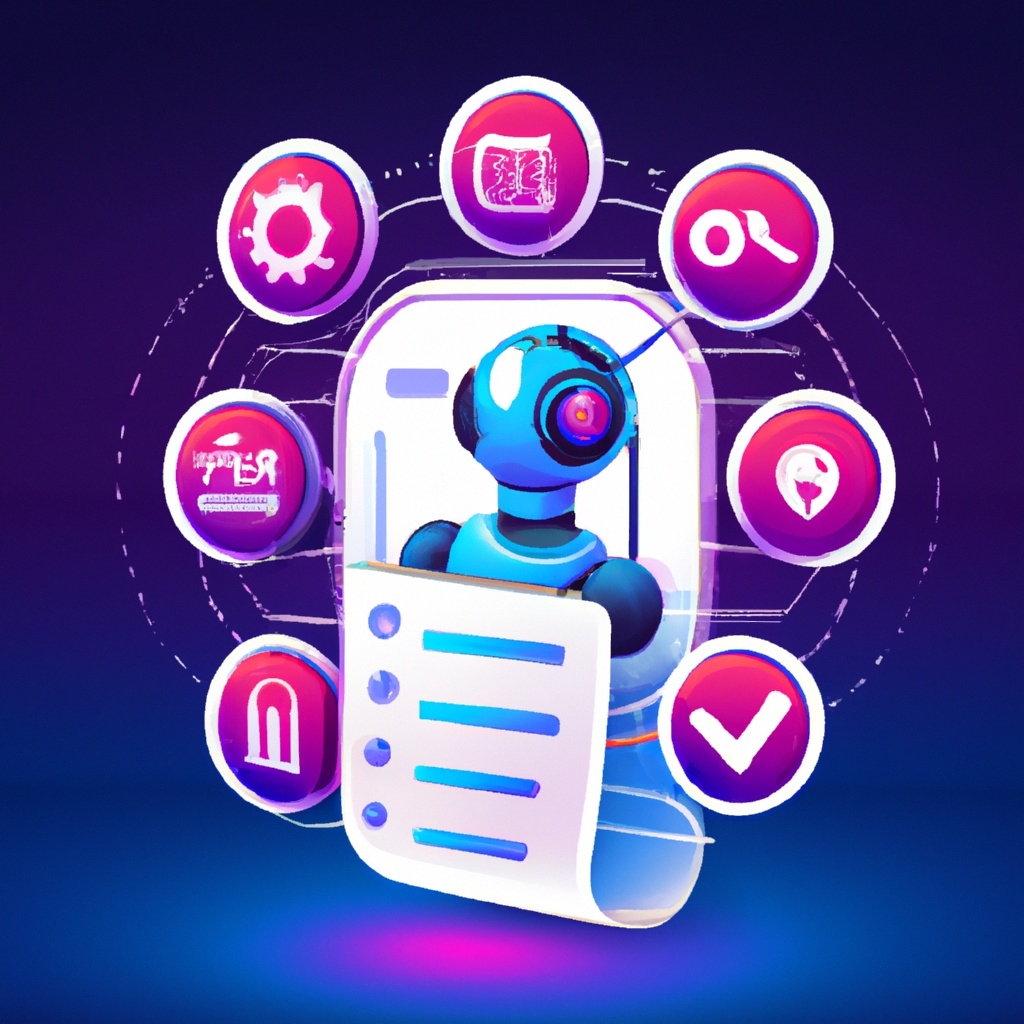
There is growing evidence of both positive and negative implications of AI chatbot technology. For every Klarna customer service success story, there are one or more Air Canada situations where incorrect information leads to fines and reputational damage. Such examples highlight both the transformative nature of AI and the importance of stewardship, especially within regulated industries.
Before rushing headlong into deploying AI, especially in customer-facing roles, companies need to invest in appropriate AI usage policies, frameworks, and architecture—as well as train people to better understand how AI is changing the way they work.
The opportunities are compelling but, explains Akber Datoo, CEO and Founder of D2 Legal Technology, to mitigate the risks associated with AI, it is vitally important to build the skills and knowledge to use AI legally, ethically, and effectively while maintaining data confidentiality.
Introduction
AI has taken the world by storm over the past 18 months. With the advent of Open AI’s ChatGPT in November 2022, the potential and promise of generative AI was suddenly within the reach of every individual and every organisation. Yet despite the phenomenal adoption of AI technologies, there remains an almost wilful misunderstanding of the associated risks – risks that are considerable, especially for organisations operating within regulated industries.
Indeed, the sheer ease of use of these tools is further undermining the risk perception. Organisations need to understand enough about how these tools operate to be able to adequately utilise them in a safe manner with appropriate processes cognisant of their limitations and risks. How many have assessed the implications for regulatory compliance, including data privacy (e.g. GDPR, CCPA), Know Your Customer (KYC) and Anti-Money Laundering (AML)? Or recognised the vital importance of well–governed and appropriate–quality data to deliver effective, accurate and trustworthy output?
These issues are just the start when it comes to creating a robust corporate AI strategy. Organisations are rushing headfirst to deploy AI chatbots not only internally but in customer facing roles, without even considering the fact that they may have no right to use the AI output due to IP ownership issues. Or assessing the different risk postures associated with developing an in-house tool versus using a commercial option, not least the implications for data confidentiality and associated risk of a compliance breach. Organisations clearly want to explore the potential business optimisation and cost efficiencies available but where is the legal understanding to mitigate the very significant risks?
Mixed Messages
The temptation to accelerate AI adoption is understandable. There is no doubt that AI has the potential to deliver substantial operational benefits. Klarna’s AI assistant, for example, was able to handle two-thirds of customer service chats in its first month – some 2.3 million conversations which would all previously have been handled by humans. The implications are significant: not only is this the equivalent work of 700 full-time human agents, but customer satisfaction levels were (at least) on par with those of human agents. The company achieved a 25% drop in repeat inquiries due to greater accuracy in errand resolution, a nine-minute improvement in errands resolution, and an estimated additional $40 million USD in profit.
However, for every good news AI story, there are multiple instances of AI providing incorrect or inconsistent information. TurboTax and H&R Block have faced recent criticism for deploying chatbots that give out bad tax-prep advice, while New York City has been compelled to defend the use of an AI Chatbot amid criticism and legal missteps following the provision of incorrect legal advice to small businesses. Even more high profile was the case where Air Canada’s chatbot gave a traveller incorrect advice – advice which was upheld by the British Columbia Civil Resolution Tribunal, which then insisted the airline pay damages and tribunal fees. The chatbot’s advice had conflicted with information available elsewhere on the airline’s website, and there was important discussion around where the liability rests when a company enlists a chatbot to be its agent.
These experiences are likely just the tip of the iceberg in terms of both good and bad AI generated outcomes. Indeed, market analyst Gartner’s Strategic Planning Assumptions include a prediction that “by 2027, a company’s generative AI chatbot will directly lead to the death of a customer from bad information it provides.”
Endemic AI Misperceptions
Given the sophisticated risk management and compliance processes in place within any regulated organisation, the big question is why are so many rushing ahead in deploying AI chatbots without either understanding the technology or undertaking a robust risk assessment? The answer is likely that the relative ease of use and the massive perceived efficiencies are causing people to proceed with changes they do not fully understand when dealing with such unfamiliar systems, without knowing how to think it through. Without in depth understanding of how the AI technology works, it will be impossible for organisations to determine how and where to deploy AI in a way that adds value and mitigates the risk appropriately.
The Air Canada – and other – examples highlight the issue of AI generating hallucinations – but do organisations understand why AI is prone to such behaviour? Generative AI is non-deterministic, which means: ask the same question in sequence and the answer could well be different. Plus, models exhibit drift: not only are they changing constantly based on the ever-growing depth of training information but the AI is learning on the job.
In a legal context, for example, AI is bad at finding citations and tends to make up fictitious citations when trying to justify the answer to a question. There is no truth or falsehood embedded within the parameter weighting, the simple fact is that the underpinning Natural Language Models (NLM) have a fundamental disadvantage when it comes to factual information. The AI does not understand the content it is generating, in the same way that a calculator does not know that it is producing numbers.
Understanding Business Implications
The implications of this disadvantage when it comes to business problem solving were highlighted in a recent study undertaken by BCG Henderson Institute. Using 750 of the Institute’s consultants, the study revealed that when using generative AI (OpenAI’s GPT-4) for creative product innovation, a task involving generating new business plan ideas and content creation, around 90% of the participants improved their performance. Further, they converged on a level of performance that was 40% higher than that of those working on the same task without GPT-4.
In contrast, when the consultants used the technology for business problem solving, they performed 23% worse than those doing the task without GPT-4. Even worse, participants who were warned about the possibility of wrong answers from the tool during a short training session did not challenge its output – underlining the misperception and false sense of security created by the apparent simplicity of such tools. Organisations need to invest in robust training that ensures individuals have an in-depth understanding of AI and, critically, continue to update their knowledge in a fast-changing environment.
These findings also underline the need to put a human in the loop. There is no traceability with AI, and no explainability as to how it operates or how output has been generated. Nominating an individual to be responsible for ensuring that nothing inappropriate, inaccurate or incorrect is provided is a fundamental aspect of any AI development.
Techniques to Mitigate AI Chatbot Risks
That said, while the risks associated with AI chatbots are real and require careful mitigation strategies, a number of approaches can (if used correctly), be used to enhance the accuracy and performance of chatbots – particularly when combined with the addition of the human in the loop.
These include:
- Fine-Tuning: By adapting a pre-trained language model to a specific domain or task, fine-tuning customises a chatbot’s behaviour and responses, making it more suitable for specific use cases.
- Retrieval Augmented Generation (RAG): This approach enhances large language models (LLMs) by incorporating a human-verified knowledge base into the response generation process. RAG dynamically pulls information from specified data sources, leading to more accurate and relevant chatbot interactions.
- Function Calling: This refers to the ability of a language model to interact with and utilise external tools or APIs (Application Programming Interfaces) to perform specific tasks. Complementing RAG with function calling enables precise queries to external databases, further optimising response accuracy and relevance.
Conclusion
Growing numbers of organisations are warning about the danger of unmanaged AI chatbots. The Consumer Financial Protection Bureau has warned the increased use of chatbots in the banking sector raises the risks – such as non-compliance with federal consumer financial protection laws, diminished customer service and trust and potential harm to consumers.
The onus is on organisations, therefore, to take a far more robust approach to understanding the technology, the evolving legal debates and risk perception. To truly unlock AI’s value, it is imperative to understand the different iterations of AI technology, determine appropriate use cases, identify robust data sources and assess the correct postures. Critically, individuals at every level of the business need to truly understand the difference between how AI could and should be used within a regulated industry.